Streaming Data into Your Lakehouse: Introducing Pulsar’s Lakehouse Tiered Storage
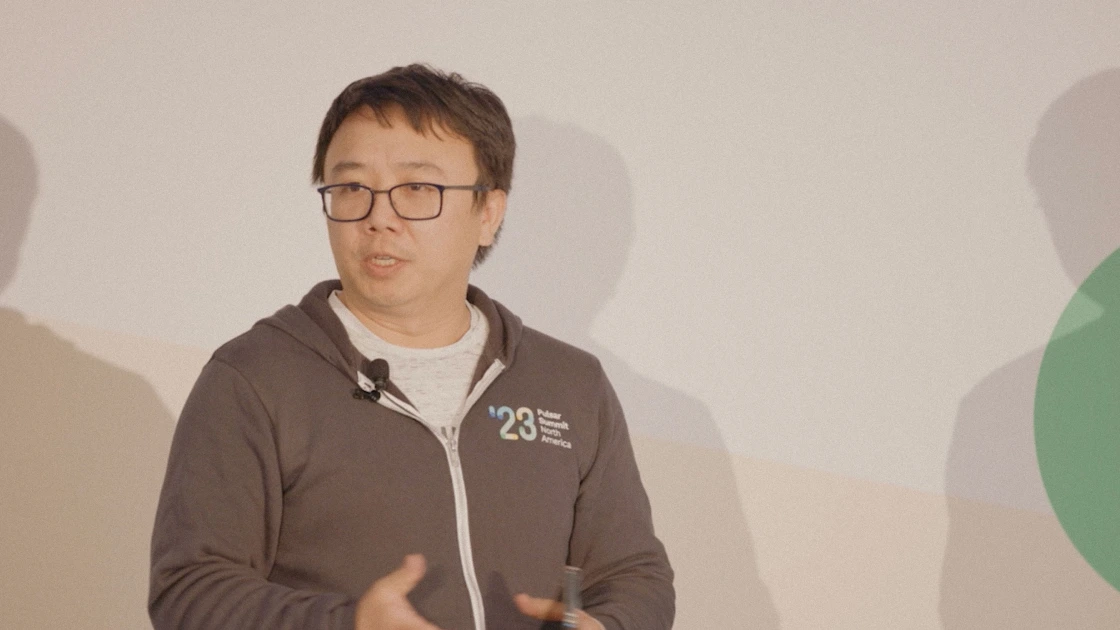
In the last 5 years, with the rise of streaming data and the need for lower latency access to data, the limits of data lakes have been reached. Lakehouse architectures, a term coined by Databricks and implemented via Delta Lake, as well as other technologies like Apache Hudi and Apache Iceberg, have quickly grown in usage and bring the following features to data lakes, such as streaming ingest of data, tools for dealing with schema and schema evolution, improved metadata management and open-standards to ease integration across a range of data processing systems.
Apache Pulsar is a distributed, open-source pub-sub messaging and streaming platform for real-time workloads. Integrating Apache Pulsar with Lakehouse will empower data lifecycle management and data analysis. We developed the Lakehouse tiered storage, which empowers Apache Pulsar as a Lakehouse. It can run within or outside of the Pulsar broker to support streaming offload topic data to Lakehouse products, such as Detla Lake, Iceberg, and Hudi with open formats. It also supports both streaming read and batch-read messages from Lakehouse. With the integration of Lakehouse tiered storage, Pulsar can support both streaming reading and batch reading effectively by routing cold data read requests to the Lakehouse which makes Pulsar more competitive in data analysis.
In this talk, we will introduce the Lakehouse tiered storage, deep dive into the details, and demonstrate how we can integrate this with other streaming query engines.
This Session recording was originally presented at Pulsar Summit North America 2023.
Newsletter
Our strategies and tactics delivered right to your inbox